NVIDIA® has dropped an exciting new update to their existing Jetson Orin™ product line just in time for the holiday season. It is called the super mode, which boosts the internal clocks of the NVIDIA® Jetson Orin™ Nano and NX to increase AI performance and memory throughput further.
Disclaimer: The software to boost performance for Jetson Orin NX and Orin Nano modules will be released in January 2025.
Fig 1: Details of Jetson Commercial Modules Performance with Super Mode (Source: NVIDIA®)
Users can expect to see up to 1.7 times improvement (depending on the AI model and other system conditions) in their AI workloads with their existing system with just a software update.
Fig 2: Performance Improvement of NanoOwl ViT Model with Super Mode On (Source: NVIDIA®)
NVIDIA® provides a software update to Jetson Orin Nano Super developer kit. We tried to benchmark the increased performance that is possible with a small test setup running the YOLOv8n model for object detection on an Orin™ Nano running JP 5.1.2 vs. JP 6.1 with super mode enabled. The test results will be updated with more details later.
These are the configuration details of test case 1:
- Device: NVIDIA® Orin™ Nano dev kit
- OS: Jetpack 5.1.2 (L4T 35.4.1)
- Power mode: 15W
- Demo App: Object Detection using the YOLOv8n model
- Model input resolution: 384×640
- Camera used: See3CAM_CU81 running at 1280×720 (8MP HDR Camera)
This is what we observed:
- Average Inference time: 21.3ms
- Minimum inference time: 19.2ms
- Maximum inference time: 23.1ms
Fig 3: Screenshot Showing JTOP and Camera Preview with YOLO Object Detection Running in Real Time
Next, we updated the same system to JP 6.1.2 and modified the power mode as follows:
- Device: NVIDIA® Orin™ Nano dev kit
- OS: Jetpack 6.1 (with super mode on)
- Power mode: MAXN
- Demo App: Object Detection using YOLOv8n model
- Model input resolution: 384×640
- Camera used: See3CAM_CU81 running at 1280×720
These are our observations with super mode enabled in a more complex scene:
- Average Inference time: 18.3ms
- Minimum inference time: 17.2ms
- Maximum inference time: 19.3ms
That is about a 3ms reduction in average inference time on a more complex scene. All these test results are without any other optimizations done and only with supermode on. With further optimization techniques such as TensorRT, we can get even more performance from the same system.
Fig 4: Screenshot with JTOP, Inference Time, and Camera Preview with YOLO Running in Realtime with Super Mode On
With the increased bandwidth and AI computing of existing kits, users can process more frames per second from their existing camera setup and systems. Now that’s a great Christmas gift for your team. Happy computing!
Disclaimer: These are preliminary test results. This blog will be updated later with more comprehensive details.
For further queries, please write to us at camerasolutions@e-consystems.com
Don’t forget to check our Camera Selector portfolio for NVIDIA Jetson compatible cameras.
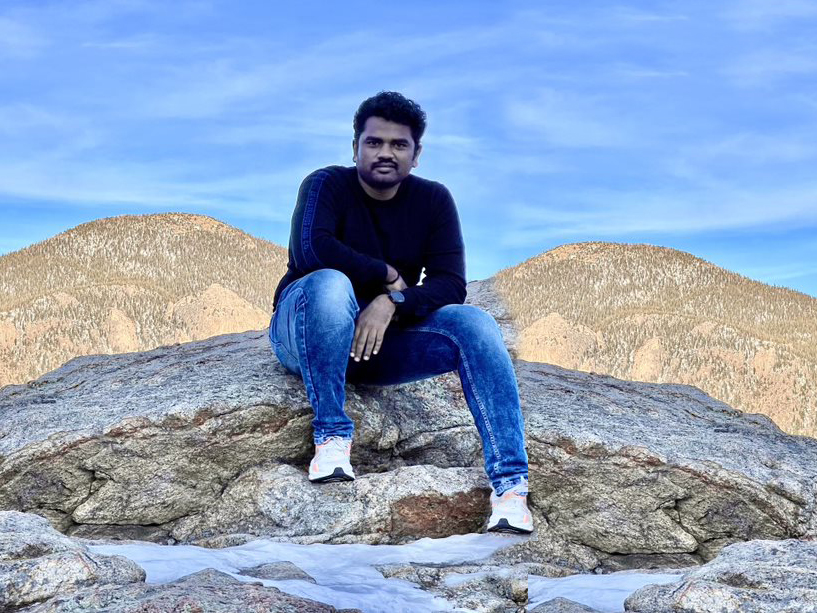
Gomathi Sankar is a camera expert with 15+ years of experience in embedded product design, camera solutioning, and product development. In e-con Systems, he has built numerous camera solutions for robots, industrial handhelds, quality inspection systems, smart city applications, industrial safety systems, and more. He has played an integral part in helping hundreds of customers build their dream products by integrating the right vision technology into them.